Professor Andy Tatem recently spoke at a virtual event on Geospatial for sustainable agriculture and urban development in Africa organised by The Food and Agriculture Organization of the United Nations (FAO). In this recording of his presentation, Professor Tatem gives an overview of our work and shares examples of the impact of our collaborations with UN agencies and national statistic offices in Afghanistan, Burkina Faso, Nigeria and the Democratic Republic of Congo, and highlights our work supporting national census preparation, health programmes and disaster response in low- and middle-incomes countries globally.
“We're trying to tackle these kinds of situations where data
that we trust may be available but at course resolution, or data that exists is
outdated or incomplete or inaccurate.”
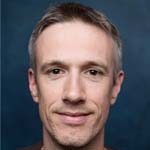
Top-down tutorials
- Small area population estimates using random forest top-down disaggregation: An R tutorial
- Small area population estimates using random forest top-down disaggregation
Bottom-up tutorial
See also
- Multi-temporal age/sex structured subnational census/projections
- Worldpop – Population Data Portal
- Moving beyond 1979: Afghanistan
- Census completion: Burkina Faso
- Supporting health intervention campaigns: Nigeria
- Vaccination and survey planning: Democratic Republic of Congo
- WorldPop Open Population Repository (WOPR)
References
- Boo et al (2022) – High-resolution population estimation using household survey data and building footprints
- Leasure et al (2020) – National population mapping from sparse survey data: A hierarchical Bayesian modeling framework to account for uncertainty
- Pezzulo et al (2021) – Geographical distribution of fertility rates in 70 low-income, lower-middle-income, and upper-middle-income countries, 2010–16: a subnational analysis of cross-sectional surveys
- Sorichetta et al (2016) – Mapping internal connectivity through human migration in malaria endemic countries
- Jochem et al (2019) – Identifying residential neighbourhood types from settlement points in a machine learning approach
- Jochem et al (2020) – Classifying settlement types from multi-scale spatial patterns of building footprints
- Dooley et al (2021) – WorldPop Open Population Repository (Buildings)
- Lloyd et al (2020) – Using GIS and Machine Learning to Classify Residential Status of Urban Buildings in Low and Middle Income Settings
- Wardrop et al (2018) – Spatially disaggregated population estimates in the absence of national population and housing census data
- Linard et al (2013) – Modelling spatial patterns of urban growth in Africa
- Wang et al (2022) – Projecting 1 km-grid population distributions from 2020 to 2100 globally under shared socioeconomic pathways
- The Value of Modelled Population Estimates for Census Planning and Preparation